Afshin Karimi
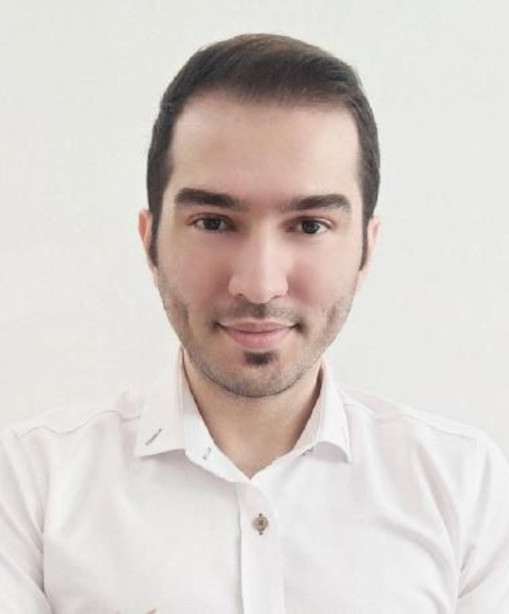
DESY
Notkestraße 85 (Building 25B)
22607 Hamburg
Room: 121
AI-Powered XFEL Laser Operations: Boosting Uptime with Language Models
Supervisors: Dr. Henrik Tünnermann (DESY), Dr. Ingmar Hartl (DESY), Prof. Anne Lauscher (UHH)
Afshin has a strong academic and professional background in Artificial Intelligence, Natural Language Processing (NLP), and Machine Learning. He completed his Master’s degree in Computer Engineering at Sharif University of Technology, where he specialized in AI and Data Science. His research focused on financial time series prediction, leading to a publication in the Journal of Supercomputing on Bayesian-based classification for trend prediction.
Professionally, he has worked as an AI/NLP Engineer, contributing to various projects involving large-scale data processing, information extraction, and language model optimization. His experience includes fine-tuning large language models (LLMs) for real-world applications, integrating retrieval-augmented generation (RAG) techniques, and working with graph-based methods like Microsoft GraphRAG and HippoRAG to enhance LLM performance. He has also worked extensively with Elasticsearch, Kafka, and ray.io for building scalable AI-driven pipelines.
His research interest lies at the intersection of LLMs, knowledge graphs, and retrieval-augmented generation (RAG). He is particularly interested in optimizing knowledge retrieval for LLMs using graph-based approaches to improve efficiency and accuracy.
For his PhD, he aims to advance the integration of knowledge graphs with LLMs to improve structured reasoning and information retrieval. His goal is to explore novel ways of encoding semantic relationships within graph structures to support more accurate and factually consistent LLM outputs.
At DESY, he looks forward to working on multimodal data processing and AI-driven knowledge extraction in collaboration with his supervisors. His expertise in deep learning, NLP, and knowledge graphs aligns well with the research objectives of the institute, and he is eager to contribute to projects that bridge the gap between LLMs and structured data representations.