Prof. Jörg Behler
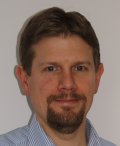
Zoom Meeting &
On-Site at the DESY, Building 99 / CFEL, Seminar Room II (Registration Necessary: https://indico.desy.de/e/dsc14)
November 24th, 2022
2 pm
Chair: Prof. Matthias Rarey
Investigating Solid-Liquid Interfaces Using High-Dimensional Neural Network Potentials
A lot of progress has been made in recent years in the development of machine learning potentials (MLPs) for atomistic simulations [1]. While the first generation of MLPs relying on single feed-forward neural networks has been restricted to small molecules with only a few degrees of freedom, the second generation extended the applicability of MLPs to high-dimensional systems containing thousands of atoms by constructing the total energy as a sum of environment-dependent atomic energies. High-dimensional neural network potentials (HDNNP) [2] have been the first example of a second-generation MLP, and to date HDNNPs have been applied to many different types of systems. Long-range electrostatic interactions can be included in third-generation HDNNPs employing environment-dependent charges [3], but only recently limitations related to the underlying locality approximation could be overcome by the introduction of fourth-generation HDNNPs [4], which are able to describe non-local charge transfer and multiple charge states using a global charge equilibration step. In this talk, the basic concepts of the different generations of HDNNPs will be outlined, followed by a discussion of applications with a focus on solid-liquid interfaces [5].
[1] J. Behler, J. Chem. Phys. 145 (2016) 170901.
[2] J. Behler, M. Parrinello, Phys. Rev. Lett. 98 (2007) 146401.
[3] N. Artrith, T. Morawietz, J. Behler, Phys. Rev. B 83 (2011) 153101.
[4] T. W. Ko, J. A. Finkler, S. Goedecker, J. Behler, Nature Comm. 12 (2021) 398.
[5] M. Eckhoff, J. Behler, J. Chem. Phys. 155 (2021) 244703