Deep Learning for X-Ray Phase Contrast Tomography
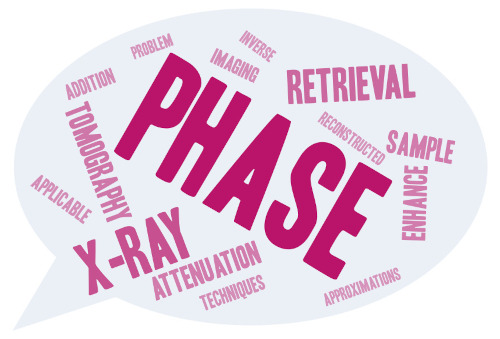
Deep Learning for X-Ray Phase Contrast Tomography
Supervisors: Prof. Martin Müller (Hereon), Dr. Julian Moosmann (Hereon), Prof. Timo Gerkmann (UHH)
X-ray imaging techniques such as radiography and computed tomography have various applications in medicine, industry, and science. Conventional X-ray imaging relies on the attenuation of X-rays traversing the sample. It thus fails to visualize very weakly or homogeneously attenuating materials such as soft tissues (as known from medical CT). Here, phase-contrast X-ray tomography is employed, which exploits local phase variations of the X-ray wavefront exiting the sample. In addition, phase contrast can enhance weak signals, e.g., in data from in situ or in vivo experiments where X-ray doses or scan times are critical. In any case, an additional data processing step is required before tomographic reconstruction. The so-called phase retrieval typically relies on certain approximations that are not applicable in the case of many samples. Then, phase retrieval is an ill-posed inverse problem as phase and attenuation must be reconstructed from a single intensity measurement.
This problem is related to phase retrieval in speech enhancement, where, in the past years, machine learning techniques have been employed, considerably pushing the field forward. In this project, the expertise in speech enhancement and X-ray tomography are combined to advance both fields. In particular, we explore deep learning-based approaches, e.g., using generative adversarial networks, to solve the inverse of phase retrieval beyond commonly applied approximations.
Requirements:
- Master's degree in computer science, physics, or electrical engineering
- proficient in Python and familiar with modern libraries for machine learning
- experience in signal processing and/ or computational Imaging
Position:
- Helmholtz-Zentrum Hereon
- 100% EGR. 13 (TV-AVH) position for three years, pending approval of funding
Hereon offers flexible working hours and work-life balance opportunities and promotes equal opportunities and diversity. In principle, the full time position (39 h/week) is also sharable.
Hereon promotes equal opportunities and diversity. The professional development of women is very important to us. Therefore, we strongly encourage women to apply for the vacant position.
Applications from severely disabled persons will be given preference if they are equally qualified.